AI products – table of contents:
Introduction to AI products management
AI products require constant development and customization, which is different from traditional technology solutions.
- AI, artificial intelligence – a general name for the ability of machines to perform tasks that mimic the workings of human reason and creativity, such as recognizing images, understanding written and spoken language, or making decisions based on available data,
- ML, machine learning – a sub-discipline of AI covering processes in which machines learn from data and experience how to perform tasks better. The uniqueness of machine learning (ML)-based products comes from the fact that they are not pre-programmed, but are equipped with learning and adaptation capabilities. In industries such as healthcare, AI contributes to more precise diagnostics, while in finance it enables more sophisticated risk analysis,
- GenAI, generative artificial intelligence – a new field of ML involving systems that can create new content, such as text, images, video, 3D models or music, based on the user’s invention or user-specified purpose and input data such as keywords, queries, or prompts, or sketches or photos.
AI product planning – from idea to implementation
Planning an AI product requires asking a key question at the outset: Will this product benefit from adding AI capabilities?
Implementing an AI product is risky and expensive, and as a result, it’s a good idea to start by defining the problem to be solved by the AI implementation, and then try to solve it optimally. Perhaps using brainstorming with ChatGPT or Google Bard, which can surprisingly advise on the optimal product development path – not necessarily based on AI.
However, if we decide to add artificial intelligence to a company’s offerings, we need to consider the specifics of the AI project life cycle. After all, Gartner data shows that only 54% of AI projects make it from the pilot phase to production.
This is often due to the very promising prototypes that can be created with the AI tools available today. On the other hand, it is very difficult to achieve “production quality” and the repeatability and relevance of results required by stakeholders.
The AI product life cycle differs from others, however, not only in that it goes beyond the concept phase somewhat less frequently. Where the life cycle of traditional products tends toward a gradual decline in interest once sales peak, AI products experience the so-called “flywheel effect.” This is a phenomenon in which a machine learning-based product improves as it is used and new data is collected from users. The better the product is, the more users choose it, which in turn generates more data to improve the algorithm. This effect creates a feedback loop that enables continuous improvement and scaling of AI-based solutions.
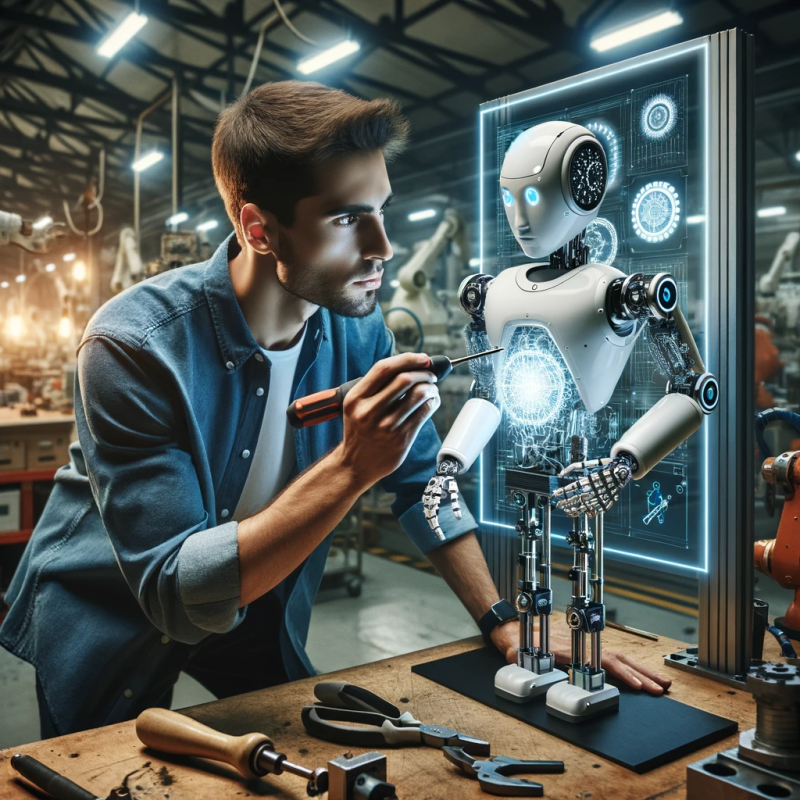
Source: DALL-E 3, prompt: Marta M. Kania (https://www.linkedin.com/in/martamatyldakania/)
This makes them products with a renewing life cycle. In other words, the flywheel effect in AI means that continuous improvements lead to incremental improvements in product performance. For example:
- Iterative training of AI models – for example, a model for sales forecasting may require repeated training to achieve optimal accuracy, but it becomes more and more perfect over time,
- Data backlog management – for content personalization applications, collecting and analyzing user data can be a priority, which will gradually lead to more and more relevant results.
In summary, AI project management requires flexibility and readiness for continuous improvement. Therefore, AI project managers must be prepared to meet changing requirements and constantly adjust strategies.
Understanding data and its role in AI product development
The role of data in AI product development is crucial. McKinsey estimates that generative AI models could generate economic benefits of up to $4.4 trillion annually. However, reaching for a piece of that pie requires quality data management.
For example, for an e-commerce product recommendation system to work well, the quality of customer behavior data is crucial. Not only will you need the right amount of data, but also its proper segmentation and updating, and most importantly, skillful drawing of conclusions from the collected information.
When creating a data-driven AI product, it is equally important to maintain impartiality in the data. For example, in AI algorithms used in recruitment or insurance, the data mustn’t contain implicit biases – based on gender or location – that could lead to discrimination.
It is worth noting that proper data management requires not only technical expertise but also awareness of its impact on the performance of AI products.
The most common problems when managing AI-based products
Managing AI products involves challenges that require specific skills and ethical awareness. Among the most important problems are worth mentioning:
- AI skills development – for example, a product manager in the AI industry needs to understand the basics of machine learning to work effectively with the technical team,
- up-to-date orientation to legal requirements – regulations on AI products are just emerging, so you need to be oriented to adjust your company’s policies and regulations for using the AI product on an ongoing basis,
- integrating AI into existing systems – integrating advanced artificial intelligence into existing IT systems can pose technological and organizational challenges,
- scaling AI solutions – for technology start-ups, developing an AI prototype into a full-scale product requires resources, time and expertise, which can also be a problem due to the relatively low supply and high demand for specialists,
- keeping users engaged – for an app that uses AI to personalize content, constantly adapting to users’ changing preferences is key to keeping them engaged,
- addressing ethical dilemmas – for example, in an AI application for health monitoring, privacy and security of user data is a priority.
AI products – summary
In summary, managing AI projects and products requires an understanding of the unique challenges and opportunities that the technology brings. Understanding the role of data, being able to manage teams and projects as well as staying aware of the ethical aspects of AI are the essential. AI products are opening new horizons for business, but they require the right approach and skills.
For start-ups, it is important to focus on clearly defining the problem the AI product is meant to solve and building a team with the right knowledge and experience in AI. It’s also worth focusing on building ethical and transparent AI systems that comply with user expectations and regulations.
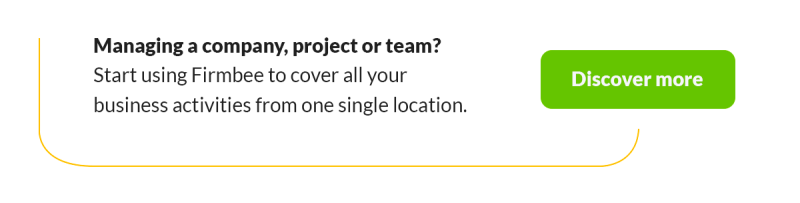
If you like our content, join our busy bees community on Facebook, Twitter, LinkedIn, Instagram, YouTube, Pinterest, TikTok.
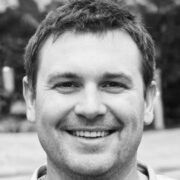
Author: Robert Whitney
JavaScript expert and instructor who coaches IT departments. His main goal is to up-level team productivity by teaching others how to effectively cooperate while coding.
AI in business:
- Threats and opportunities of AI in business (part 1)
- Threats and opportunities of AI in business (part 2)
- AI applications in business - overview
- AI-assisted text chatbots
- Business NLP today and tomorrow
- The role of AI in business decision-making
- Scheduling social media posts. How can AI help?
- Automated social media posts
- New services and products operating with AI
- What are the weaknesses of my business idea? A brainstorming session with ChatGPT
- Using ChatGPT in business
- Synthetic actors. Top 3 AI video generators
- 3 useful AI graphic design tools. Generative AI in business
- 3 awesome AI writers you must try out today
- Exploring the power of AI in music creation
- Navigating new business opportunities with ChatGPT-4
- AI tools for the manager
- 6 awesome ChatGTP plugins that will make your life easier
- 3 grafików AI. Generatywna sztuczna inteligencja dla biznesu
- What is the future of AI according to McKinsey Global Institute?
- Artificial intelligence in business - Introduction
- What is NLP, or natural language processing in business
- Automatic document processing
- Google Translate vs DeepL. 5 applications of machine translation for business
- The operation and business applications of voicebots
- Virtual assistant technology, or how to talk to AI?
- What is Business Intelligence?
- Will artificial intelligence replace business analysts?
- How can artificial intelligence help with BPM?
- AI and social media – what do they say about us?
- Artificial intelligence in content management
- Creative AI of today and tomorrow
- Multimodal AI and its applications in business
- New interactions. How is AI changing the way we operate devices?
- RPA and APIs in a digital company
- The future job market and upcoming professions
- AI in EdTech. 3 examples of companies that used the potential of artificial intelligence
- Artificial intelligence and the environment. 3 AI solutions to help you build a sustainable business
- AI content detectors. Are they worth it?
- ChatGPT vs Bard vs Bing. Which AI chatbot is leading the race?
- Is chatbot AI a competitor to Google search?
- Effective ChatGPT Prompts for HR and Recruitment
- Prompt engineering. What does a prompt engineer do?
- AI Mockup generator. Top 4 tools
- AI and what else? Top technology trends for business in 2024
- AI and business ethics. Why you should invest in ethical solutions
- Meta AI. What should you know about Facebook and Instagram's AI-supported features?
- AI regulation. What do you need to know as an entrepreneur?
- 5 new uses of AI in business
- AI products and projects - how are they different from others?
- AI-assisted process automation. Where to start?
- How do you match an AI solution to a business problem?
- AI as an expert on your team
- AI team vs. division of roles
- How to choose a career field in AI?
- Is it always worth it to add artificial intelligence to the product development process?
- AI in HR: How recruitment automation affects HR and team development
- 6 most interesting AI tools in 2023
- 6 biggest business mishaps caused by AI
- What is the company's AI maturity analysis?
- AI for B2B personalization
- ChatGPT use cases. 18 examples of how to improve your business with ChatGPT in 2024
- Microlearning. A quick way to get new skills
- The most interesting AI implementations in companies in 2024
- What do artificial intelligence specialists do?
- What challenges does the AI project bring?
- Top 8 AI tools for business in 2024
- AI in CRM. What does AI change in CRM tools?
- The UE AI Act. How does Europe regulate the use of artificial intelligence
- Sora. How will realistic videos from OpenAI change business?
- Top 7 AI website builders
- No-code tools and AI innovations
- How much does using AI increase the productivity of your team?
- How to use ChatGTP for market research?
- How to broaden the reach of your AI marketing campaign?
- "We are all developers". How can citizen developers help your company?
- AI in transportation and logistics
- What business pain points can AI fix?
- Artificial intelligence in the media
- AI in banking and finance. Stripe, Monzo, and Grab
- AI in the travel industry
- How AI is fostering the birth of new technologies
- The revolution of AI in social media
- AI in e-commerce. Overview of global leaders
- Top 4 AI image creation tools
- Top 5 AI tools for data analysis
- AI strategy in your company - how to build it?
- Best AI courses – 6 awesome recommendations
- Optimizing social media listening with AI tools
- IoT + AI, or how to reduce energy costs in a company
- AI in logistics. 5 best tools
- GPT Store – an overview of the most interesting GPTs for business
- LLM, GPT, RAG... What do AI acronyms mean?
- AI robots – the future or present of business?
- What is the cost of implementing AI in a company?
- How can AI help in a freelancer’s career?
- Automating work and increasing productivity. A guide to AI for freelancers
- AI for startups – best tools
- Building a website with AI
- OpenAI, Midjourney, Anthropic, Hugging Face. Who is who in the world of AI?
- Eleven Labs and what else? The most promising AI startups
- Synthetic data and its importance for the development of your business
- Top AI search engines. Where to look for AI tools?
- Video AI. The latest AI video generators
- AI for managers. How AI can make your job easier
- What’s new in Google Gemini? Everything you need to know
- AI in Poland. Companies, meetings, and conferences