Do you know what role data analysis plays in UX research? Today, we’d like to focus on the issue of data analysis in UX by discussing qualitative and quantitative data analysis, and learning about its stages, main goals as well as objectives. We’ll also suggest when is the right moment to conduct it in a project.
Data analysis in UX research – table of contents:
- Why analyze the collected data?
- When to analyze the data?
- Data analysis in UX research
- Defining the objectives of the analysis
- Qualitative analysis of research data
- Summary
Why analyze the collected data?
Making a product decision based solely on raw data is a huge UX mistake. Skipping the analysis stage can result in providing users with an incomplete or ineffective solution, or even cause the project team to focus on solving the wrong problem or recognizing the real users. For these and other reasons, data analysis is an essential process that keeps the entire project on the right track. It does so by taking into account the real needs of users and gathering information that helps to develop the best and most optimal solution possible.
When to analyze the data?
Many people harbor a gross misconception that analysis should take place after completing the research, i.e, upon collecting information from many sources. However, this approach is ineffective, as examining such a large amount of data requires huge effort, manpower and time. It is more efficient to investigate data on an ongoing basis, for example, taking a few minutes after each in-depth interview.
Also, remember to take notes during your research. This way, you can put fresh observations down and make sure nothing is omitted. These reflections make you easily select information and choose from it those that will be most relevant for later design recommendations. Analyzing on an ongoing basis, after every little research step, allows you to conduct the final summary analysis in a far more organized and structured way, but above all, much faster.
Data analysis in UX research
Data analysis in UX research transforms previously unprocessed data into meaningful information that will support business decisions. Conducting a comprehensive data analysis consists of five basic steps – these steps are:
- Defining the objectives of the analysis
- Organizing data
- Investigation
- Clusterization
- Identification of results and insights
Defining the objectives of the analysis
The first step defines the goals of our analysis – these should come in strict accordance with the goals of UX Research. At this stage, remember not to deviate from the motives that made you set off conducting research – for example, what are the user’s needs; on which page the rejection rate is more significant and why; what improvements to make to increase the conversion rate; or how to make our product more attractive than the competition. Holding on to these, and research objectives will help you understand how to conduct data analysis in a way that is useful for the project. To define exactly what you are looking for.
Organizing data
Each survey provides different types of data, more and less relevant to the project. Thus, you have to manage, select and filter them cleverly for usability. Organizing data also enables its thoughtful arrangement to swiftly scoop for the desired information when necessary. For example, you can catalog data by the sub-page of the website to which it pertains. Segregation is key to conducting efficient data analysis and improving its visualization which makes stakeholders understand the entire process better.
Investigation
The investigation phase lies at the heart of the entire data analysis process. Its main goal consists of identifying the words, ideas or phrases that appear most frequently in users’ responses and that are most likely in line with the purpose of the analysis. This process is not just about looking for words and their synonyms, but about understanding what they mean to users in their context.
Having that figured out words and expressions means depending on the studied user group. It happens because people vary. They have unique experiences and behaviors, as well as ways of expressing themselves. Hence, you should avoid transcribing user responses to your vocabulary. Instead, stick to the original as much as possible, because any variation, even the smallest one, can harm the investigation phase reshaping the entire data analysis altogether.
Clustering
The next step is to devise so-called clusters to label the answers according to those identified in the investigation phase. These clusters help the team to differentiate prioritized issues. For example, if more than half of the user responses fit into the created cluster labeled “Interface performance,” the team should probably prioritize this topic and look for issues specifically related to interface performance.
Identification of results and insights
Let’s not forget that results aren’t insights. Results concern the discovered, investigated, then grouped and cataloged facts that the research team brought to light through the process of analysis. Insights, on the other hand, refer just to the act of recognition of causes that occasioned the results. This is quite a distinct feature since user responses do not always lead to the source of the problem. The designer’s job, then, is to look deeper and search for insights.
Users are usually unable to identify the source of their difficulties on their own. The research team must therefore review the results during the data analysis process, discuss them, and then search for insights and match them to the research objectives. A workshop to identify the most relevant insights helps accomplish this task. Effective use of this tool involves conducting several rounds of discussion separated by short breaks.
The steps described above are a fairly general and standard data analysis process that works with any research method (both qualitative and quantitative). All you need to do is properly adapt the steps to your process.
Quantitative vs. qualitative data analysis
Although the process of analyzing quantitative data, is not significantly different from analyzing qualitative data, due to the nature of this research, designers may receive different insights. Quantitative research focuses on collecting and analyzing numerical data, using statistics and probability. Indicators such as the rejection rate of a given page, for example, or the demographic profile of a user, provide researchers with concrete and quantifiable information about how people interact with the product and the audience itself.
Qualitative research focuses more on abstract concepts, such as human behavior. For this reason, take a little more time to study and evaluate to fully comprehend user experience and opinions. It’s worth asking helpful questions at this stage, such as:
- What do users like most about the product and what do they like least?
- Why do some users react differently than others?
- Did (and when) users have an emotional reaction?
- Are (and why) users satisfied with the product?
Given the difference in the data received, it makes sense to use both quantitative and qualitative anecdotes as part of UX research. That way the gathered data complement each other and give clear and deeper insight into the results.
Summary
Properly conducted data analysis allows for better, more optimal design decisions. Omitting its findings leads to developing an incomplete, ineffective product that doesn’t respond to users’ actual needs. This is why data analysis is such a critical process that determines the success of the entire project. It empowers you to collect and select key information that, when translated into concrete design recommendations, helps to develop the best possible solution – tailored to users’ needs and requirements. The data analysis steps we described will help you carry it out in a structured manner and focus on what matters most.
If you like our content, join our busy bees community on Facebook, Twitter, LinkedIn, Instagram, YouTube, Pinterest, TikTok.
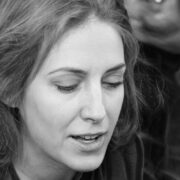
Author: Klaudia Kowalczyk
A graphic & UX Designer which conveys into design what cannot be conveyed in words. For him, every used color, line or font has a meaning. Passionate in graphic and web design.
UX research:
- What is UX research?
- Types of UX research
- What are research questions and how to write them?
- Requirements gathering process for UI/UX projects
- Why are stakeholder interviews crucial for the design process?
- How to leverage our gathered customer data?
- How to create a good UX research plan?
- How to choose a research method?
- How can pilot testing improve UX research?
- UX study participant recruitment
- Channels and tools for finding UX research participants
- Screener survey for UX Research
- UX Research Incentives
- UX research with children
- Discovery research methods
- What is desk research?
- How to conduct user interviews?
- How to conduct diary studies?
- What are focus groups in research?
- What is ethnographic research?
- Survey research
- What is card sorting in UX?
- What is evaluative research?
- How to conduct usability testing?
- When and how to run preference testing?
- What is A/B testing in UX?
- Eyetracking in UX testing
- What is tree testing?
- First click testing
- What is task analysis in UX research?
- Evaluation of emotions in UX
- Continuous Research in UX
- Data analysis in UX research
- How to prepare a UX research report?
- Customer Journey Map – what is it and how to create it?